Uncovering the 10 Biggest Flaws in Generative AI
- 25 May 2023
- 477
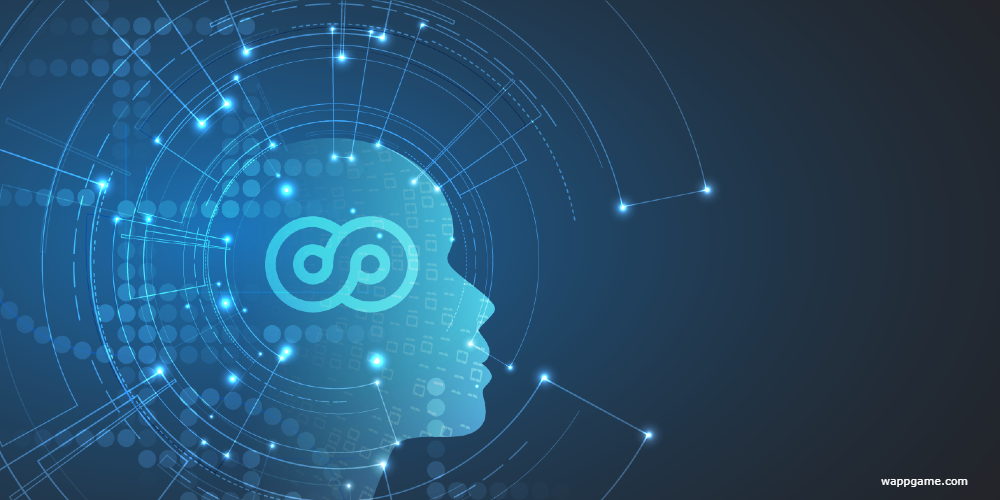
As technology advances, artificial intelligence (AI) is becoming more commonplace and taking on an increasingly important role in our daily lives. One of the most promising branches of AI is "generative AI" – algorithms that enable artificial systems to learn by example and produce unique outputs. While this may sound like a revolutionary advancement, there are several inherent flaws that users need to be aware of.
1. Bias in Training Data
One of the most significant flaws in generative AI lies in the potential for biased training data. If the dataset contains inherent biases – whether in terms of gender, race, or any other factors – then the AI model will likely produce biased results. This can lead to skewed conclusions, unfair representation or treatment of certain groups, and potentially even discrimination.
2. Model Overfitting
A common issue encountered by generative AI is overfitting – a phenomenon where an AI model becomes too attuned to its training data and consequently performs poorly with new information. When a model is overfitted, it may appear highly accurate during training but will struggle to generalize beyond that specific dataset.
3. Inability to Understand Context
Current generative AI models still struggle to fully comprehend context when creating content or responding to inputs. While they can generate seemingly coherent text or images from a statistical perspective based on their training dataset patterns, they often lack a genuine understanding of underlying meanings and can misinterpret nuances.
4. Ethical Concerns
Ethical concerns frequently arise in relation to generative AI applications such as deep fake videos or misleading content creation. These technologies have the potential for malicious use or disinformation dissemination, leading some experts to call for stricter regulations and guidelines surrounding their use and development.
5. Inaccurate Content Generation
While generative AI can produce impressive outputs in certain conditions, there are instances where it might generate misleading or inaccurate content. This flaw can negatively impact data analysis, decision-making processes, or even user experience when relying on AI-generated information.
6. Duplication and Plagiarism
Generative AI models are highly capable of producing content akin to existing sources, which can be problematic regarding originality and plagiarism. This issue not only compromises the intellectual property rights of original creators but also raises concerns about the overall creativity and innovation of AI-generated material.
7. Lack of Transparency
A major challenge with generative AI is the inherent lack of transparency, sometimes referred to as the "black box" problem. Opaque algorithms may become less trustworthy as users cannot easily understand their decision-making process or comprehend what factors influenced specific conclusions.
8. Resource Intensity
The training and deployment of generative AI models often require substantial computational resources and energy consumption. These costs may limit accessibility for smaller organizations or researchers lacking significant resources, ultimately hindering broader development and innovation in the field.
9. Difficulty in Control
An ongoing challenge with generative AI is fine-tuning control over its outputs without sacrificing creativity or autonomy. In some cases, this might involve using mechanisms like reinforcement learning to achieve desired outcomes while maintaining a balance between human control and algorithmic flexibility.
10. Legal Implications
The rapid evolution and adoption of generative AI have led to a myriad of legal questions concerning copyright ownership, defamation claims, human rights issues (e.g., privacy concerns), and more. As technology advances, lawmakers and regulators will need to grapple with these complex legal implications.
In conclusion, despite the many achievements of generative AI, it is crucial to recognize the various shortcomings of the technology. Identifying these shortcomings allows us to develop more ethical, innovative, non-infringing solutions that will ultimately move AI and its applications even further into the future.